Revolutionizing GPU Resource Automation
Introducing the industry’s first AI-augmented GPU workload automation platform.
Rapt's AI-augmented platform continuously observes workloads to determine optimal model resources, identifies and optimizes the right GPU resources in real-time and orchestrates closed loop automation to match optimal resources.
Our Trusted Partners:
Our Numbers Say It All
10X
More workloads on same infrastructure
ZERO
Infra Set-up and Tuning Time
70%
Reduction in GPU infrastructure costs
95%
GPU utilization
The Critical Role of GPU Workload Automation
Enhancing Efficiency and Scalability Throughout the AI Lifecycle
-
A GPU workload automation refers to the process of optimizing and managing the execution of computationally intensive tasks on GPUs. This involves efficiently allocating GPU resources, scheduling jobs, and monitoring performance to maximize utilization and productivity across the AI development lifecycle.
-
GPUs are integral part of AI success. AI engineers have to integrate GPUs into AI workflow of data preparation, to feature engineering, model development, training and deployment. GPU automation ensures that GPUs are available at all times, used effeciently with continuous resouce monitoring to make sure AI workflows run smoothly with no delay, disruptions and low cost.
-
Successful GPU workload automation platforms include components like workload management, resource allocation, scheduling, monitoring, and optimization. Each of these elements is crucial for maximizing performance, reducing costs, and handling the dynamic and unpredictable nature of AI workloads.
-
AI workloads are dynamic and unpredictable. Understanding your specific workload characteristics in real-time is critical in provisioning right GPU resources. Any preset resource configurations leads to disruptions in model runs, high latencies and inefficient over provisioned resources.
-
Accessing GPUs across clouds and on-premise is a huge challenge. Due to the difficulty, on premise or in the cloud, fully utilizing your existing GPUs is also difficult. This limited availability, and high costs, leads to managing complex multi-hybrid clouds and GPU sourcing. This complexity forces organizations and Data Scientists to wait for these expensive resources.
-
Assessing your infrastructure to determine what is the right configuration, whether on-premise or cloud-based or hybrid deployment is suitable. In addition you need to consider; evaluate with trial-error methods, estimations to configure GPUs and GPU shares, network bandwidth, and memory.
-
Balancing costs with capabilities is essential. Significant time is spent by Data Scientists and ML engineers tuning the infrastructure for each individual workload. In addition, Ops/IT teams are not privy to the model characteristics that impact and provision the ideal infrastructure. This leads to over provisioned, static, locked-in resources, exceeding AI compute budgets, while also resulting in limited GPU availability for others.
-
Existing workload orchestration tools like Kubernetes offer workload orchestration without any insights into GPU usage, no resource optimizations, no dynamic resourcing for changing dynamic and unpredicatable models, no fault-tolerence in case of spot disruptions, and node failures. Complex management leads to siloed, locked-in cloud and on-prem GPUs.
Essential Considerations for GPU Workload Automation
Unlocking Unparalleled Efficiency and Performance
How Rapt Stands Out Among the Competition
Rapt vs. Cloud-based Platforms
While cloud platforms like AWS, Azure, and Google Cloud offer GPU-powered instances with basic management tools, Rapt provides advanced AI-driven optimization and auto-fractional sharing. This allows for more granular control and better cost-efficiency compared to traditional cloud-based solutions.
Rapt vs. On-premise Platforms
On-premise solutions such as Kubernetes and DCGM offer control over GPU resources but require significant technical expertise. Rapt simplifies this process with a user-friendly interface and automated resource management, reducing the complexity of on-premise deployments while enhancing performance.
Key Advantages of Rapt
Rapt's AI-driven resource prediction, multi-cloud support, and cost-aware scheduling make it a superior choice for organizations looking to optimize their AI infrastructure. Unlike other platforms, Rapt combines ease of use with powerful optimization features, ensuring maximum GPU utilization and cost savings.
Real-World Applications
Unlocking Efficiencies in BioTech AI Workflows
A multinational Fortune 100 pharmaceutical company faced a critical need to get data science results faster without increasing their spend on infrastructure. They developed an AI platform for hundreds of data scientists specializing in LLM AI models for drug discovery models. The company's AI infrastructure included public cloud GPU instances, on-premises NVIDIA DGX servers, and over 300 data scientists spread across multiple continents.
However, they encountered several critical challenges :
-
Time-consuming trial-and-error setup and dynamic model infra requirements, OOM errors, and guesswork plagued model training.
-
Static GPU allocations led to over-provisioning and underutilization.
-
Cloud and on-premises silos caused scare and PU resources.
-
Challenges in managing data privacy and data gravity.
-
One-size-fits-all model configurations lacked flexibility.
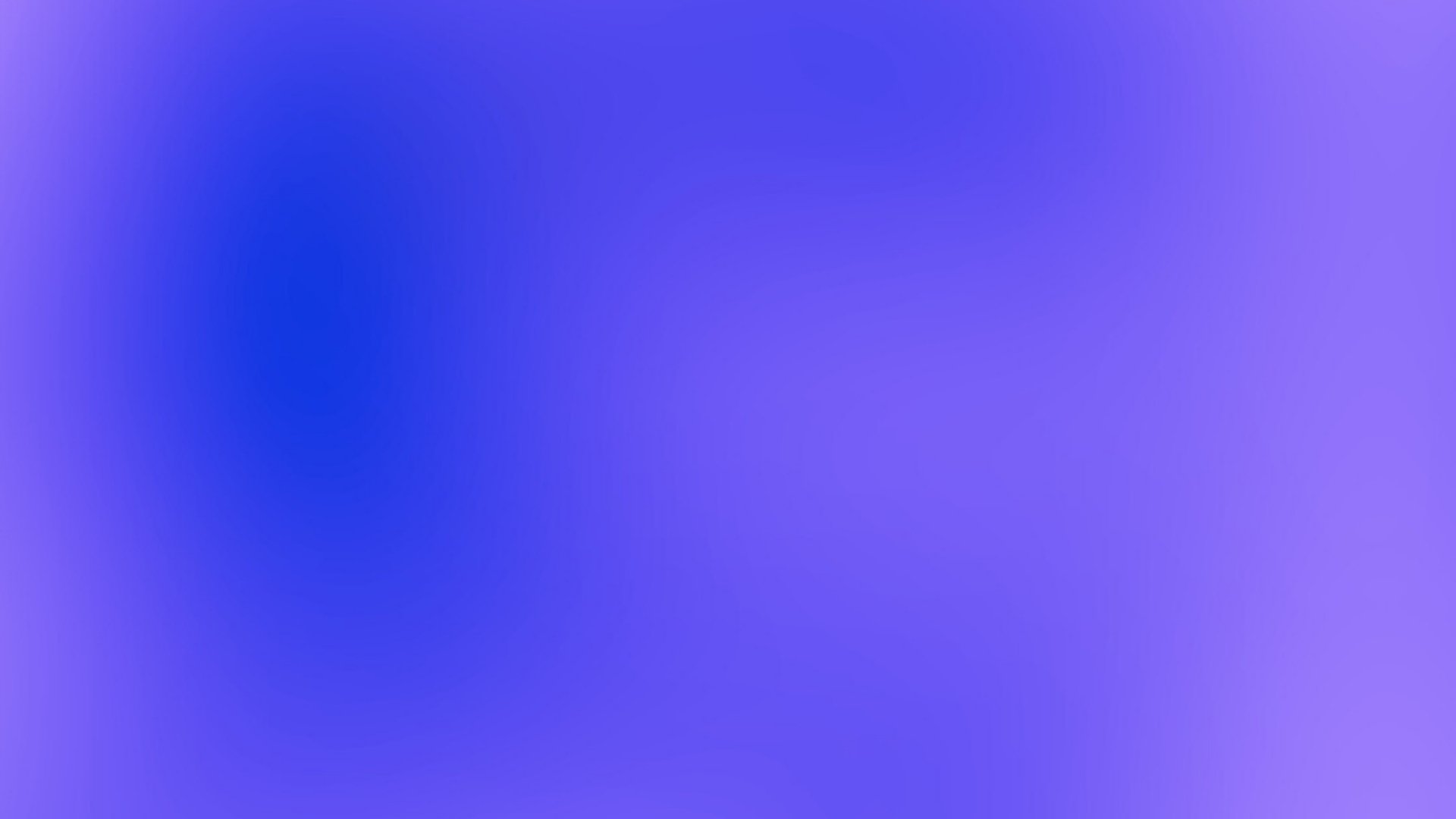
TESTIMONIAL
"The Rapt platform allows our Data Scientists to run Al models with one-click. This eliminates infra setups and resource configurations, increasing productivity by at least 4x. They can also run 3x more models in the same infrastructure and we pay 70% less to cloud while maximizing our on-premise Al servers."
- Global Life Sciences | Sr. Manager, Al Platforms
Transform Your AI Infrastructure.